November 27, 2023 at 5:19 pm | Updated November 27, 2023 at 9:46 pm | 6 min read
The search for quality in apple production is perpetual. The research paper by Biegert et al., titled “Modelling Soluble Solids Content Accumulation in ‘Braeburn’ Apples,” offers a pivotal step forward in this search. Published in the journal Plants, this study leverages optical sensor data to transform our understanding of apple sweetness—quantified by the Soluble Solids Content (SSC)—without the need for traditional, invasive testing methods for apple quality prediction.
This post is designed not merely as a narrative of their study but as an exploratory dialogue that extends the research’s reach, delving into the balance of light and chemistry that defines the essence of apple quality. Here, we look at the study’s methodology, innovative use of non-destructive optical measurements, and the insights garnered.
Objectives of SSC Investigation
The researchers’ primary goal was to establish a model that accurately reflects the accumulation of SSC in ‘Braeburn’ apples—a key determinant of fruit quality that affects the taste and ensures commercial viability. This investigation into the world of apple sweetness sought to answer pivotal questions: How can we predict the SSC in a way that informs better harvest times and storage practices? Why is it crucial to understand this metric within apple’s maturation journey?
Subscribe to the Felix instruments Weekly article series.
By submitting this form, you are consenting to receive marketing emails from: . You can revoke your consent to receive emails at any time by using the SafeUnsubscribe® link, found at the bottom of every email. Emails are serviced by Constant Contact
The study aimed to look past the barriers of traditional SSC assessment methods, replacing them with a non-invasive, optical sensing approach. By doing so, the researchers aspired to enhance the scientific community’s ability to monitor and manage fruit quality in a way that aligns with the industry’s move towards more precise and sustainable agriculture. Through this article, we look into why these objectives are not just scientifically significant but also essential for the perpetual evolution of apple production practices.
The Context of SSC in Apple Quality
Apple quality stems from environmental conditions, orchard management, and the intrinsic biology of the fruit. SSC is a crucial indicator of the fruit’s flavor and storability. This measure of sweetness has traditionally been a predictor of consumer satisfaction and market success. However, the challenge has been to measure it without resorting to destructive methods that reduce the sellable yield.
The research community has put forth efforts to shift from intrusive to non-destructive testing, making the study by Biegert et al. particularly timely. It mirrors the industry’s broader shift towards precision horticulture, where understanding the nuances of SSC can lead to better crop management and postharvest quality. The research is anchored in the reality of this shift, offering insights that could revolutionize apple quality assessment and storage practices.
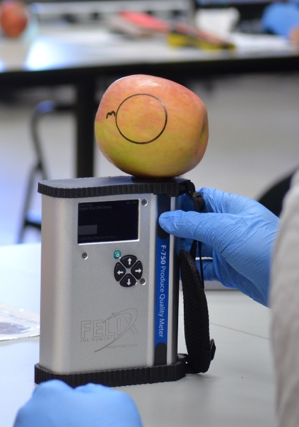
Practical Implications of SSC Insights
The practical ramifications of this research many parts of the orchard, offering tangible benefits to the apple industry. By accurately predicting SSC, farmers and fruit producers can better determine the optimal harvest time, ensuring that the apples reaching consumers are at their peak of flavor and quality. This insight directly impacts the marketability and economic return of the produce. Moreover, the findings from this study have significant implications for postharvest management—informing storage practices to extend the shelf life of apples and reduce waste.
The non-destructive testing methods developed through this research could become a cornerstone of modern precision agriculture, enabling more responsive and sustainable orchard management. The ability to monitor SSC non-invasively allows for larger datasets, providing a more nuanced understanding of how different factors affect apple sweetness. This granular level of detail could lead to advancements in selective harvesting, where fruit is picked based on quality markers rather than a uniform harvest date, paving the way for a new era of tailored agriculture. The applications extend beyond the immediate field of horticulture; the methodologies and insights from this study can inform a range of agricultural practices and policies, fostering an environment where quality, sustainability, and profitability grow hand in hand.
Study Results: Seed Viability in Different Conditions
The research conducted over three distinct apple cropping seasons in Southwest Germany yielded insightful data, emphasizing the dynamic nature of agricultural environments. 2016, 2017, and 2018 brought unique challenges, from wet springs and frost events to a notably hot and dry year, significantly influencing plant development and fruit characteristics. The study meticulously tracked soluble solids content (SSC) and fruit diameter growth, revealing a consistent SSC accumulation trend toward apple maturity despite some variability due to environmental factors. Notably, the highest SSC values were observed in 2018, with less precipitation and warmer conditions.
The Partial Least Squares Regression (PLSR) calibration models, used to predict SSC from hyperspectral data, showed root mean square errors of prediction (RMSEP) between 0.54% and 0.67% across the three years, indicating a reliable performance of the models. The multi-year model demonstrated reasonable predictions with minor systematic errors, suggesting a dependable method for estimating SSC over variable conditions. However, the research also revealed that models calibrated on yearly data tended to overfit and performed poorly when applied to data from other years, indicating the necessity for robust multi-year models.
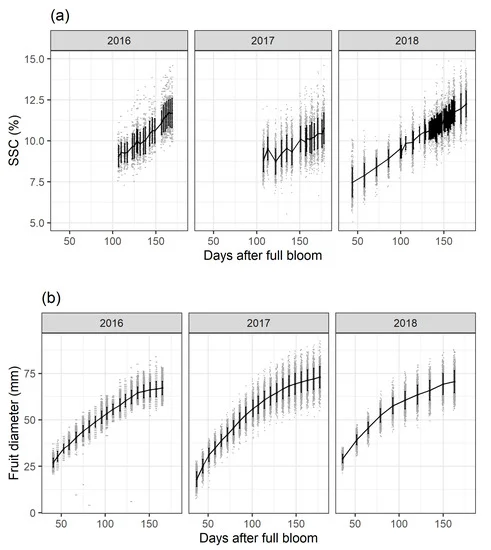
Innovative Tools for Smarter Agriculture
The F-750 Produce Quality Meter supported this complex research, contributing to the larger data pool with its hyperspectral imaging capabilities. The technology behind the F-750 allowed for non-destructive, real-time data acquisition, contributing to the comprehensive datasets required for developing the PLSR models. The study’s findings underscore the importance of such advanced tools in research, where data precision and methodological reliability are paramount.
The study’s exploration into the effects of different calibration sample sizes and the robustness of models against laboratory errors illustrated the practicality and resilience of the PLSR method. Even when subjected to potential laboratory errors, the PLSR models maintained their robustness, a testament to the stability of the technology used. Furthermore, the comparison between spectral and conventional laboratory methods to determine SSC at harvest demonstrated that while the non-destructive, technology-driven approach showed a higher variance, it did not bias the results, offering a viable alternative to traditional destructive testing methods.
These insights serve not only to validate the use of instruments like the F-750 but also to pave the way for their broader application in precision agriculture. Such technologies, capable of delivering real-time, non-destructive data, are invaluable in agricultural research and beyond.
Challenges Ahead in Seed Science
The study candidly acknowledges certain limitations, which open avenues for further investigation. While the PLSR models proved robust, slight systematic errors were indicated, with the possibility of underestimating or overestimating extreme SSC values, especially for the years 2017 and 2018. Moreover, the yearly calibrated models, although precise within their respective years, were less transferable to other years, suggesting that each growing season’s unique conditions can dramatically affect the model’s applicability.
The heteroscedastic structure of residuals points to varying prediction accuracy across the SSC range, with less reliability at the extreme ends of the spectrum. Furthermore, the models’ performances were intrinsically linked to the range of SSC values in the calibration data, which in turn was influenced by the environmental variances of each year.
Future research is suggested to focus on enhancing the PLSR models’ transferability across different years and environmental conditions. Investigations could explore integrating additional spectral and ecological data to bolster the models’ resilience to annual variability. Also, expanding the calibration data set to include a broader range of conditions and SSC values could mitigate the overfitting observed in year-specific models.
Additionally, future studies may delve into the implications of reduced sample sizes and the potential of simplifying data collection without compromising the accuracy of predictive models. The prospect of integrating these non-destructive technologies into routine agricultural practices calls for additional research into cost-effective and scalable applications.
Essential Takeaways
- The research illustrated the profound impact of environmental conditions on apple SSC accumulation, with significant yearly variations.
- PLSR models developed from hyperspectral imaging data provided reliable SSC predictions with minimal errors, emphasizing the potential of non-destructive testing in agriculture.
- Calibration models are sensitive to annual climatic variations, underscoring the need for multi-year models to improve robustness.
- Systematic errors were minimal but present, indicating room for refinement in model accuracy, especially for extreme SSC values.
- Non-destructive spectral methods, including using the F-750, demonstrated viability as alternatives to traditional destructive testing despite higher variance.
- Sample size and laboratory errors influence PLSR model performance, yet models remained robust even under simulated laboratory inaccuracies.
- The research contributes to the body of knowledge supporting advanced technologies in precision agriculture, with potential implications across various agricultural practices.
- Future research directions include improving model transferability, expanding calibration datasets, and exploring cost-effective implementation for practical agricultural use.
The study conducted over varying seasonal conditions has showcased the resilience and potential of hyperspectral imaging and PLSR models in determining the soluble solids content of apples—a critical quality parameter in agriculture. It has affirmed the value of non-destructive testing methods, such as those enabled by the F-750 Produce Quality Meter, in providing accurate, real-time data crucial for advancing agricultural research and practices. While acknowledging its limitations, the study opens promising pathways for future exploration, particularly in enhancing model transferability and efficiency across diverse environmental scenarios.
Sources
Biegert, K.; Stöckeler, D.; McCormick, R.J.; Braun, P. Modelling Soluble Solids Content Accumulation in ‘Braeburn’ Apples. Plants 2021, 10, 302. https://doi.org/10.3390/plants10020302
Related Products
Most Popular Articles
- Spectrophotometry in 2023
- The Importance of Food Quality Testing
- NIR Applications in Agriculture – Everything…
- The 5 Most Important Parameters in Produce Quality Control
- Melon Fruit: Quality, Production & Physiology
- Liquid Spectrophotometry & Food Industry Applications
- Ethylene (C2H4) – Ripening, Crops & Agriculture
- Guide to Fresh Fruit Quality Control
- Active Packaging: What it is and why it’s important
- Understanding Chemometrics for NIR Spectroscopy